Open Data
Particle Flow Reconstruction
Scalable Neural Network Models and Terascale Datasets
One of the main approaches for event reconstruction at the Large Hadron Collider (LHC) currently relies on particle flow (PF), which combines hits across subdetectors, considering the full event to reconstruct all stable particles in the event. Given the planned High-Luminosity (HL) LHC program, as well as possible future experimental programs of e.g., the Future Circular Collider (FCC), computationally efficient and physically optimal evolutions of the PF-based event reconstruction need to be developed and tested.
​
Among various approaches, there has been considerable interest and development of Machine Learning (ML)-based reconstruction methods, including for full-event reconstruction. To support rapid progress of such approaches, it is beneficial to establish open datasets with sufficient realism and granularity for testing various types of approaches.
​
In light of this, we describe, and make available, an extensive open dataset of physics events with full GEANT4 simulation, suitable for PF reconstruction, available in the EDM4HEP [1] format.
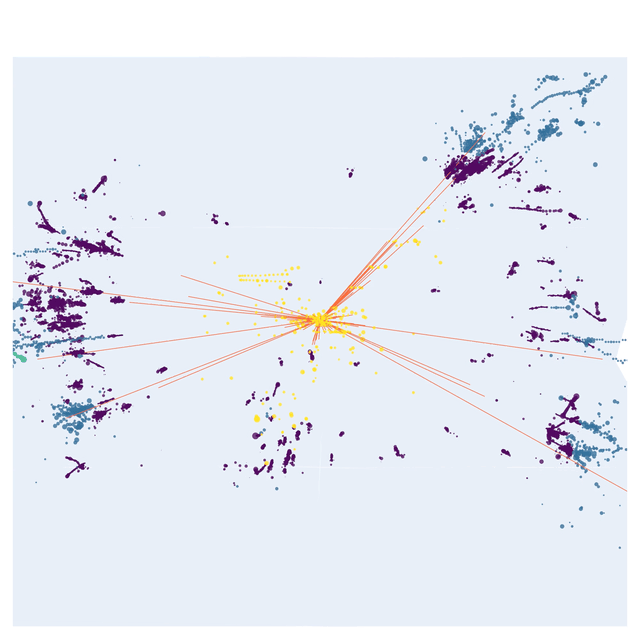
Figure 1: 3D visualization of the generator particles (targets) and the calorimeter hits in a single event.
​We generate dedicated events with Pythia8 [2] and carry out a full detector simulation with GEANT4 using the Key4HEP framework [3]. In particular, we use the CLIC detector model [4], along with the Marlin reconstruction code [5], and the Pandora [6,7,8] package for a baseline particle flow implementation. Although the implementation is not specific to the detector model, the CLIC model is chosen since, to our knowledge, it is one of the most complete publicly available realistic detector models.
Description of files and download
The datasets with all generator particles (training targets); reconstructed tracks, calorimeter hits and clusters (training inputs); as well as reconstructed particles from the baseline Pandora algorithm (for comparison) are saved in the EDM4HEP format. In addition, all associations between the aforementioned objects are saved in the standard format. Overall, the size of the dataset is approximately 2.5 TB.
This dataset is being used in studies of the Machine-Learned Particle-Flow (MLPF) algorithm [9,10,11] and new results are being prepared for publication in the near future. Any works using this dataset should cite the corresponding paper, once published.
​
The dataset consists of physical collision events as well as particle gun samples and is packaged in 43 tar archives with the naming convention <process_name>_<number>.tar for the physical samples and <process_name>.tar for the gun samples, where <process_name> refers to the name of the physics process and <number> is a running integer. Each tar archive contains ROOT [12] files where the physics events are saved in the EDM4HEP format. To process the data for ML tasks, the Python package uproot [13], which allows for convenient data loading of ROOT files into Python and NumPy objects, is recommended.
​
The datasets were generated as part of the project "Flexible and scalable data reconstruction and analysis using machine learning", grant PSG864 of the Estonian Research Council, using the KBFI computing cluster.
Dataset download:
References
[1] Gaede, F., Ganis, G., Hegner, B., Helsens, C., Madlener, T., Sailer, A., Stewart, G. A., Volkl, V., & Wang, J. (2021). EDM4hep
and podio - The event data model of the Key4hep project and its implementation. EPJ Web of Conferences, 251, 03026.
https://doi.org/10.1051/epjconf/202125103026
[2] Bierlich, C., Chakraborty, S., Desai, N., Gellersen, L., Helenius, I., Ilten, P., Lönnblad, L., Mrenna, S., Prestel, S., Preuss, C. T.,
Sjöstrand, T., Skands, P., Utheim, M., & Verheyen, R. (2022). A comprehensive guide to the physics and usage of PYTHIA
8.3. SciPost Physics Codebases, 8. https://doi.org/10.21468/SciPostPhysCodeb.8
[3] Ganis, G., Helsens, C., & Völkl, V. (2022). Key4hep, a framework for future HEP experiments and its use in FCC. The
European Physical Journal Plus, 137(1), 149. https://doi.org/10.1140/epjp/s13360-021-02213-1
[4] CLIC Collaboration. CLICdet: The post-CDR CLIC detector model. CLICdp note. 2017
[5] Gaede, F. (2006). Marlin and LCCD—Software tools for the ILC. Nuclear Instruments and Methods in Physics Research
Section A: Accelerators, Spectrometers, Detectors and Associated Equipment, 559(1), 177–180.
https://doi.org/10.1016/j.nima.2005.11.138
[6] Marshall, J. S., & Thomson, M. A. (2012). The Pandora Software Development Kit for Particle Flow Calorimetry. Journal of
Physics: Conference Series, 396(2), 022034. https://doi.org/10.1088/1742-6596/396/2/022034
[7] Marshall, J. S., Münnich, A., & Thomson, M. A. (2013). Performance of particle flow calorimetry at CLIC. Nuclear Instruments
and Methods in Physics Research Section A: Accelerators, Spectrometers, Detectors and Associated Equipment, 700, 153–
162. https://doi.org/10.1016/j.nima.2012.10.03
[8] Marshall, J. S., & Thomson, M. A. (2015). The Pandora software development kit for pattern recognition. The European
Physical Journal C, 75(9), 439. https://doi.org/10.1140/epjc/s10052-015-3659-3
[9] Pata, J., Duarte, J., Vlimant, J.-R., Pierini, M., & Spiropulu, M. (2021). MLPF: efficient machine-learned particle-flow
reconstruction using graph neural networks. The European Physical Journal C, 81(5), 381.
https://doi.org/10.1140/epjc/s10052-021-09158-w
[10] Pata, J., Duarte, J., Mokhtar, F., Wulff, E., Yoo, J., Vlimant, J.-R., Pierini, M., & Girone, M. (2023). Machine Learning for
Particle Flow Reconstruction at CMS. Journal of Physics: Conference Series, 2438(1), 012100.
https://doi.org/10.1088/1742-6596/2438/1/012100
[11] Wulff, E., Girone, M., & Pata, J. (2023). Hyperparameter optimization of data-driven AI models on HPC systems. Journal of
Physics: Conference Series, 2438(1), 012092. https://doi.org/10.1088/1742-6596/2438/1/012092
[12] Brun, R., & Rademakers, F. (1997). ROOT — An object oriented data analysis framework. Nuclear Instruments and Methods
in Physics Research Section A: Accelerators, Spectrometers, Detectors and Associated Equipment, 389(1–2), 81–86.
https://doi.org/10.1016/S0168-9002(97)00048-X
[13] Pivarski, J., Das, P., Burr, C., Smirnov, D., Feickert, M., Gal, T., Kreczko, L., Smith, N., Biederbeck, N., Shadura, O., Proffitt, M.,
Krikler, B., Dembinski, H., Schreiner, H., et al. (2021). scikit-hep/uproot3: 3.14.4 (3.14.4). Zenodo.
https://doi.org/10.5281/zenodo.4537826
​