AI for data-driven models in reacting flows
Hydrogen (Hâ‚‚) is shaping to become a major actor of the European decarbonization efforts. However, traditional power and propulsion systems are designed around fossil fuels, and intense developments are needed adapt design tools for Hâ‚‚ rapidly and meet decarbonization targets. Massively parallel solvers for reactive flows are central to many design processes, and they are the result of decades of developments. AI is a key enabler in accelerating this transition to Hâ‚‚.
​
Turbulent reactive flows require high accuracy solvers and very compute-intensive workflows. In RAISE, the strategy is therefore to explore hybrid solvers, which leverage the domain knowledge in place and focus AI efforts on targeted components. Specifically, new subgrid-scale (SGS) models are needed accurately describe small-scale phenomena, inherent to Hâ‚‚ flames. RAISE partners will investigate data-driven approaches to SGS, notably based on deep neural networks. This includes two major axes:
​
-
Methodologies for accurate Hâ‚‚ combustion on small scales will be developed. This reliable simulator will enable the computation of many test cases, producing a significant dataset. Treatment of this dataset will result in databases representative of the SGS modeling task. Partners will then be able to train machine learning algorithms on this task, optimize the various strategies, and compare to existing physics-based techniques.
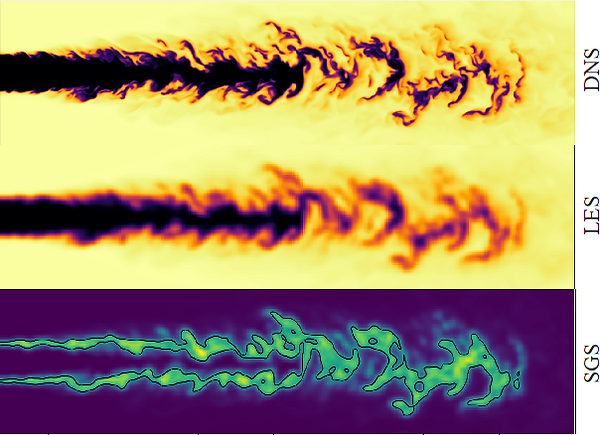
Figure 1:
Fully resolved physics in a DNS (top), equivalent lower resolution simulation achievable in an industrial LES (middle), and necessary SGS model to compensate for unresolved physics (bottom)
​
-
The AVBP-DL prototype solver, developed to explore hybrid fluid solvers with both traditional and DL methods, will be used by partners as a platform to explore problems related to hybrid computation and optimization for modern HPC clusters. Previously optimized SGS models will be implemented in this solver, and run at scale on large compute clusters to demonstrate the technology and validate the learning strategies for SGS.

Figure 2: Flame resolved using AVBP-DL, on 2000 CPUs and 128 GPUs (each GPU domain has a unique color),
using the Jean Zay supercomputer of IDRIS
This use case bears strong links with the smart models for next-generation aircraft engine design use-case. Hâ‚‚ combustion regimes will be set according to this use-case, and the resulting AVBP-DL solver with data-driven SGS model will be applied to a real-world engine simulation through it.
​
CERFACS
42 avenue Gaspard Coriolis
31057 Toulouse CEDEX 01
email: lapeyre [@] cerfacs.fr